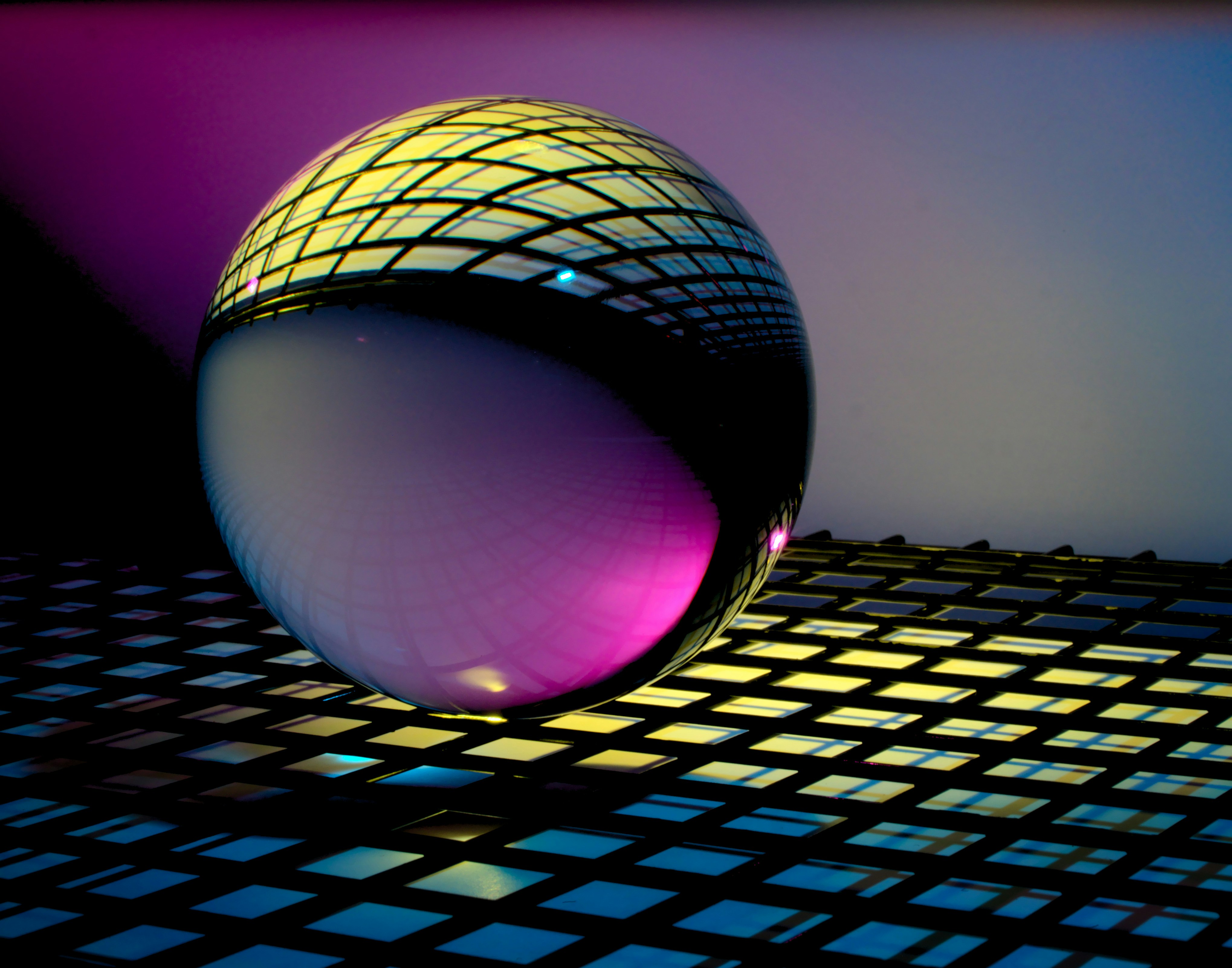
Edge Computing Predictions for the Next 5 Years: Technological Progress, Emerging Opportunities, and the Evolving Job Market
As organisations navigate the realities of high-bandwidth applications, real-time analytics, and the relentless expansion of connected devices, edge computing has emerged as a transformative paradigm—moving data storage and processing closer to the source where data is generated. From autonomous vehicles that require ultra-low latency decision-making to industrial IoT systems that manage real-time alerts on factory floors, edge computing is dramatically reshaping how businesses architect their technology. Over the next five years, the momentum behind edge computing is set to accelerate, creating both new technical frontiers and a robust job market for professionals seeking dynamic, future-facing roles.
In this comprehensive guide, we explore the key edge computing predictions over the coming half-decade, the technological progress fuelling these advances, and the career opportunities arising for UK job seekers eager to build or shift into edge-focused roles. By understanding upcoming trends—from hybrid edge-cloud deployments to AI-driven edge intelligence—you can strategically position yourself for success in an era where computing power no longer sits exclusively in remote data centres, but within devices and micro data hubs right at the network’s edge.
1. Why Edge Computing Is Poised for Rapid Growth
1.1 Addressing Latency, Bandwidth, and Data Overload
While cloud computing offers scalable infrastructure, sending enormous data volumes from distributed sensors, cameras, or connected vehicles to central data centres can introduce:
Latency Constraints: Real-time tasks—like collision avoidance in autonomous driving or sub-millisecond feedback loops in robotics—demand near-instantaneous processing.
Bandwidth Bottlenecks: Constantly streaming raw sensor data to the cloud can saturate networks, hike up costs, or run into intermittent connectivity issues.
Data Overload: Many edge-generated data points are ephemeral or only valuable in real-time, rendering continuous full-resolution cloud uploads inefficient.
By processing data locally—on or near the devices—edge computing minimises round-trip delays, reduces internet traffic, and ensures critical decisions remain uncompromised by network interruptions.
1.2 Convergence with 5G, AI, and IoT
Edge computing rarely operates in isolation. It thrives within a broader technology ecosystem:
5G: Ultra-fast, low-latency mobile networks enabling edge deployments for AR/VR, telemedicine, or collaborative robotics without performance lags.
AI and ML: Offloading inference tasks from central servers to local edge nodes, preserving bandwidth and delivering immediate insights (e.g., anomaly detection, object recognition).
IoT: Billions of sensors, wearables, and devices capturing real-world data, sending aggregates or processed signals to the cloud or directly orchestrating local actions.
This synergy allows organisations to develop advanced solutions—like real-time analytics dashboards or AI-enabled autonomous systems—that rely on distributed intelligence. For job seekers, it means edge specialists must also grasp AI frameworks, connectivity standards, and cloud integration.
1.3 Business Imperatives and User Demand
The push for edge computing is not just technological but also business-driven:
Cost Savings: Reducing cloud data egress fees by trimming raw data transmissions, only sending relevant insights upstream.
Resilience: Guaranteeing local functionality even when internet connections degrade or become unavailable.
Privacy/Compliance: Keeping sensitive data locally processed to meet GDPR or other regulations, minimising risk of mass data breaches.
Customer Experience: Providing frictionless, responsive interactions—e.g., voice assistants that respond instantly or AR overlays in retail—without the lag of cloud round-trips.
As these motivations deepen, so too does the appetite for professionals who can architect, deploy, and secure edge infrastructures that fit each organisation’s unique operational environment.
2. Edge Computing Predictions for the Next Five Years
2.1 Hybrid Edge-Cloud Solutions Become Standard
Prediction: By 2028, hybrid models—where on-premises edge nodes handle critical, time-sensitive tasks while cloud platforms coordinate global orchestration—will define mainstream deployments across the UK.
Key Drivers
Operational Necessities: Some workloads demand local processing (robotics, AR) for reliability, while others benefit from cloud-based analytics or backup.
Data Lifecycle Management: Storing raw data locally short-term, uploading summaries or aggregated results to the cloud for historical analysis.
Flexible Resource Sharing: Seamlessly moving data or compute tasks between local edge and centralised cloud as usage spikes or cost structures shift.
Implications for Job Seekers
Hybrid Orchestration Skills: Mastering container management (Kubernetes, K3s), event-driven pipelines, or open-source frameworks bridging edge devices with cloud.
Connectivity Setup: Implementing secure links (VPN, SD-WAN) between corporate data centres and remote edge nodes.
Resource Scheduling: Deciding which tasks run locally (real-time inference) and which go to the cloud (ML training), balancing latency, cost, and power constraints.
2.2 Proliferation of AI at the Edge
Prediction: Edge devices will increasingly integrate on-device AI, enabling real-time predictions, anomaly detection, or computer vision without relying on round-trips to the cloud.
Key Drivers
ML Hardware Acceleration: AI-specialised chips (GPUs, TPUs, FPGAs) or dedicated neural engines in edge devices, boosting inference speed.
Model Compression: Techniques like quantisation, pruning, and knowledge distillation letting large neural nets run on resource-limited microcontrollers.
Privacy and Bandwidth: Minimising data transfers by processing user data locally, only sending aggregated or anonymised info to the cloud.
Implications for Job Seekers
Edge AI Specialist: Merging ML frameworks (TensorFlow Lite, PyTorch Mobile) with embedded software or FPGA design.
Data Pipeline Integration: Designing flows where partial insights come from local edge inference, while aggregated data informs central analytics or retraining.
Model Ops: Devising methods to update or retrain edge models securely, ensuring consistent improvements in inference accuracy.
2.3 Edge as Key Enabler for Autonomous Systems
Prediction: Autonomous vehicles, drones, and robots—especially in manufacturing or logistics—will increasingly rely on edge nodes for real-time decision-making, shedding reliance on stable cloud connectivity.
Key Drivers
Ultra-Low Latency Demands: Industrial robotic arms or UAV swarm coordination often requires sub-10ms response times.
Connectivity Constraints: Many operational environments—factory floors, remote farmland, or maritime routes—lack reliable high-bandwidth connections.
Safety-Critical Tasks: Minimising cloud-based single points of failure or latencies that compromise crucial manoeuvres.
Implications for Job Seekers
Embedded Control: Designing real-time control loops, sensor fusion, or onboard computing for navigation or object manipulation.
Resilience and Failover: Handling partial connectivity or fallback routes (local mesh networks), ensuring safe operation if external comms drop.
Hardware-Software Co-Design: Integrating sensors, actuators, and edge processors, often under strict power or weight constraints.
2.4 Rise of Edge Data Marketplaces and Ecosystems
Prediction: As companies gather real-time data at the edge, new marketplaces or data-sharing ecosystems will emerge—facilitating on-device or local data trades, micropayments, and AI model exchanges.
Key Drivers
IoT Data Explosion: Billions of sensors generating local observations—climate data, traffic patterns, production metrics—potentially valuable to partners if shared securely.
Distributed Ledger and Smart Contracts: Potential for blockchain-based micropayments, ensuring trust in data provenance or usage rights.
Collaborative Analytics: Multiple stakeholders pooling local data or model updates for more accurate AI, akin to federated learning or on-chain data oracles.
Implications for Job Seekers
Data Governance: Understanding how to handle data ownership, privacy, and compliance in decentralised or multi-tenant edge networks.
ML Collaboration: Building federated or collaborative model training pipelines, bridging various industrial participants.
Smart Contract Integration: Creating or auditing on-chain logic that defines data exchange rules and micropayment flows, especially in cross-organisational scenarios.
2.5 Security and Zero Trust at the Edge
Prediction: With edge nodes operating outside secure data centres, adopting zero trust security models—verifying every device, user, or application continuously—will become standard.
Key Drivers
Attack Surface Expansion: Edge deployments often reside in physically exposed or semi-trusted environments (retail kiosks, city infrastructure).
Regulatory Pressure: Tighter data privacy mandates and breach notification laws demanding robust encryption, access controls, tamper-proof logs.
Threat Sophistication: Hackers targeting edge devices for lateral movement, espionage, or creating botnets.
Implications for Job Seekers
Device Identity & Access Management: Implementing hardware-based secure enclaves, certificate-based authentication, or TPM modules for device attestation.
Network Segmentation: Tools ensuring minimal privileges for each device or microservice, using software-defined perimeter concepts.
Edge-Specific Security: Roles focusing on intrusion detection at the node level, secure firmware updates, or sandboxing for local ML inference processes.
2.6 Edge Cloud Integration Tools Mature
Prediction: As hybrid edge-cloud architectures proliferate, vendor offerings—like AWS IoT Greengrass, Azure Stack Edge, or GCP Anthos—will become more robust, simplifying orchestration, monitoring, and data synchronisation.
Key Drivers
Demand for Turnkey Solutions: Enterprises wanting easy ways to connect edge compute with central analytics, device management, or DevOps pipelines.
Multi-Cloud Complexity: Tools that unify edge nodes across different providers or on-premises, providing consistent deployment frameworks.
Developer Experience: Growth in frameworks abstracting hardware differences, letting devs code once while deploying to multiple edge form factors.
Implications for Job Seekers
Vendor-Focused Skills: Familiarity with AWS IoT, Azure Edge Stack, or GCP’s edge services, plus their respective deployment and security models.
Microservices at the Edge: Container-based packaging, function-based serverless approaches, or orchestrating microservices on small-footprint devices.
Operational Monitoring: Setting up real-time metrics, logs, and remote troubleshooting for widely distributed nodes, often with sporadic connectivity.
2.7 Eco-Friendly Edge Systems
Prediction: Sustainability concerns—energy usage, e-waste, or carbon footprints—will drive greater emphasis on green edge solutions, from low-power hardware to dynamic scheduling that minimises waste.
Key Drivers
Environmental Targets: Pressure from governments, investors, or activists for net-zero or low-carbon footprints.
Remote Deployments: Edge devices in solar-powered or battery-limited contexts (rural agriculture, remote sensor networks) requiring extreme power efficiency.
Renewable Integration: Data centres or mini-hubs near wind or solar farms, offloading tasks when local energy is abundant.
Implications for Job Seekers
Low-Power Design: Knowledge of microcontroller architectures, efficient scheduling, or sensor gating for minimal energy draw.
Sustainable Lifecycle: Recyclable hardware modules, modular expansions, or software that extends device lifespans.
Cost-Energy Balancing: Roles focusing on dynamic load shifting, cost vs. carbon footprints, or policy-driven orchestration.
3. Evolving Job Market for Edge Computing in the UK
3.1 In-Demand Edge Roles
Reflecting these predictions, edge computing recruiters project growth in:
Edge Solutions Architects: Designing integrated frameworks combining local compute, connectivity, and cloud synergy.
Embedded AI/ML Engineers: Deploying or optimising neural nets for on-device inference, balancing memory, power, and accuracy constraints.
Edge DevOps / Platform Engineers: Managing container-based edge clusters, CI/CD for distributed nodes, real-time monitoring under intermittent connectivity.
IoT/Edge Security Specialists: Implementing zero trust at scale, dealing with hardware enclaves, secure key provisioning, and intrusion detection in remote conditions.
Edge Data Engineers: Crafting pipelines that unify local streaming analytics with aggregated cloud data lakes or real-time dashboards.
3.2 Core Technical Skills
Technical:
IoT and Networking: Protocols (MQTT, CoAP), 5G/6G knowledge, network security, edge node provisioning.
Distributed Systems: Understanding concurrency, partitioning, and load balancing in resource-limited environments.
Containerisation: Docker, Kubernetes (or K3s) for orchestrating microservices on constrained hardware.
Real-Time OS and Embedded: RTOS fundamentals, memory constraints, concurrency, or direct sensor interfacing.
Security Best Practices: TLS, zero trust frameworks, device attestation, robust identity management.
Soft Skills:
Collaboration: Partnering with hardware designers, data scientists, or domain experts (e.g., manufacturing line managers).
Communication: Explaining edge ROI, justifying latency minimisation approaches, or clarifying device lifecycle plans.
Resilience: Troubleshooting device failures, debugging concurrency issues, iterative design under tight constraints.
Ethical Orientation: Balancing user privacy, data minimisation, or sustainability considerations with performance goals.
3.3 Certifications and Education
Certifications:
AWS (IoT Core, Greengrass), Azure (IoT Hub, Edge), GCP (IoT Core) or vendor-neutral IoT security programmes for device provisioning and management.
Edge DevOps courses focusing on container orchestration in constrained environments or real-time constraints.
Cybersecurity credentials emphasising hardware trust, encryption, or secure embedded design.
Hands-On Experience:
Building personal edge projects—like a Raspberry Pi-based sensor aggregator, integrating local AI inference, then sending aggregated results to the cloud.
Internships or open-source contributions for frameworks bridging edge nodes with cloud orchestration, e.g., K3s, EdgeX Foundry.
3.4 Salary Prospects and Career Growth
Professionals bridging embedded systems with cloud DevOps or AI often command premium compensation, especially in high-stakes verticals (autonomous vehicles, critical infrastructure, healthcare). Mid-level roles can reach £50k–£70k, with senior architects or managers surpassing six figures. Career growth may involve leading platform-wide edge initiatives, pioneering new vertical solutions, or branching into consulting for multi-national IoT deployments.
4. How to Position Yourself for Edge Computing Jobs
4.1 Master the Basics of Cloud and IoT
Cloud Fundamentals: AWS, Azure, or GCP, including compute, storage, networking, security.
IoT Protocols: MQTT, AMQP, or LoRaWAN, plus device provisioning or digital twins.
Containers: Docker basics, Kubernetes or K3s for small-footprint device orchestration.
4.2 Build AI/ML or Real-Time Capabilities
Machine Learning: Deploying compressed models (TensorFlow Lite, PyTorch Mobile) on microcontrollers or edge hardware like NVIDIA Jetson.
Control Systems: For robotics or industrial tasks, knowledge of real-time scheduling, concurrency, or deterministic event loops.
Data Pipelines: Streaming frameworks that handle ingestion from sensors, immediate local analytics, and optional forwarding to central repositories.
4.3 Develop Strong Security and Zero Trust Approaches
IAM: Understand device identity provisioning, key rotation, or certificate-based authentication.
Device Hardening: Minimising OS attack surfaces, secure boot, firmware signing, intrusion detection in physically exposed devices.
Regulatory Insight: For healthcare or critical infrastructure, knowledge of industry guidelines around data storage, tamper-proof logs, or fail-safe mechanisms.
4.4 Practice Interdisciplinary Project Work
Group Projects: Combine software dev, embedded electronics, mechanical design, or domain subject matter (manufacturing, healthcare).
Hackathons/Competitions: Edge-themed events focusing on real-time challenges—like building a distributed sensor network or drone-based environment scanning.
DevOps Tools: Setting up Git-based CI/CD flows that push updates to multiple edge devices simultaneously, logging test results, or automating rollback if issues arise.
4.5 Engage in Networking and Community Building
Meetups and Summits: UK-based IoT, edge computing, or 5G events like Connected Britain, local IoT user groups, or Edge AI summits.
Online Communities: Slack channels, LinkedIn groups, or open-source projects discussing edge architecture best practices.
Contribution: Writing blog posts or tutorials about your edge lab projects, or presenting a talk on containerised microservices in a limited environment.
5. Conclusion: Embracing the Edge Revolution
Over the next five years, edge computing will advance from a promising architecture pattern to a core pillar for real-time analytics, AI-driven autonomy, and user-centric experiences across sectors. Whether it’s orchestrating thousands of industrial sensors in a factory, streaming crucial telemedicine data in remote clinics, or powering immersive AR on 5G networks, edge solutions provide the low-latency performance and resilience that centralised cloud alone can’t match.
For job seekers, the edge computing domain offers no shortage of challenges and rewards, from designing embedded AI systems to ensuring robust security at scale. By cultivating:
Technical Mastery: Cloud fundamentals, IoT protocols, AI frameworks, DevOps pipelines, and real-time embedded constraints.
Cross-Functional Collaboration: Working effectively with mechanical teams, data scientists, or product managers, bridging hardware and software.
Innovative Mindset: Seizing new vantage points for local analytics, offline functionality, or domain-specific transformations (manufacturing, healthcare, retail).
Ethical and Sustainable Approaches: Minimising environmental footprints, safeguarding user privacy, and ensuring inclusive design.
You’ll be poised to drive the next wave of distributed computing, forging solutions that transform how we interact with devices, data, and each other at the network’s edge.
Explore Edge Computing Career Opportunities
Ready to launch or elevate your edge computing career? Visit www.edgecomputingjobs.co.uk for the latest edge-focused vacancies across the UK. From DevOps specialists orchestrating multi-site container clusters to AI engineers optimising on-device inference, our platform connects you with the organisations pioneering the next frontier in distributed systems.
Seize the moment—develop your skills, forge strategic connections, and embrace the challenges of architecting real-time, resilient, and transformative edge solutions that power the era of ubiquitous compute.